AI to help optimize buildings’ power consumption
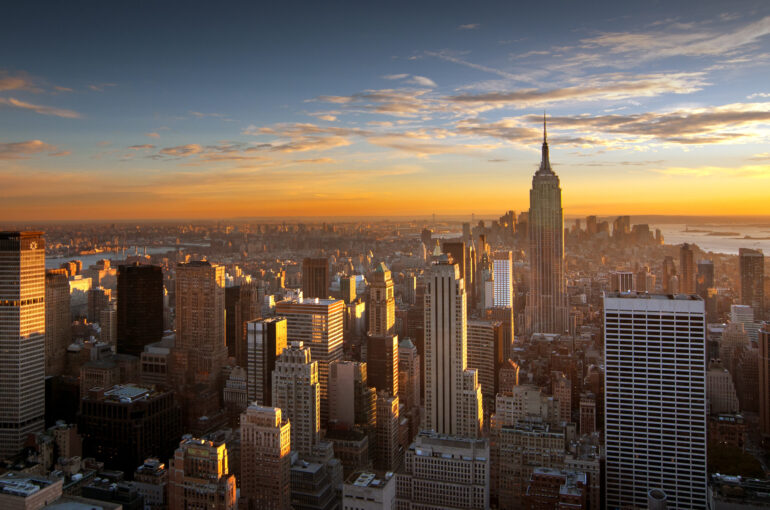
Power consumption in buildings is responsible for more than 30% of CO2 emissions. In New-York, it reaches 70%, making its skyscrapers the city’s main polluting source. As a result, how can AI technology help us reduce the carbon footprint of buildings?
To answer these questions, I met with Samuel Nadeau-Piuze – co-founder of Maxen Technology, a startup which uses AI to optimize buildings’ power usage – in Montreal, and David Hsu – Professor in environmental and urban planning at the MIT and researcher at the MIT Energy Initiative – in Cambridge, Massachusetts.
Vizualising and understanding the power consumption of a building
“When one arrives in a building, its inhabitants have, most of the time, no idea of its power consumption. At first, our job will be to make these information available” (Samuel Nadeau-Piuze). In this respect, Maxen Technology offers a platform to its customers where the power consumption of the building appears in real-time. Maxen Technology’s AI also gives the opportunity to estimate the building’s power consumption on a medium-term basis as well as creating notifications in case of abnormal consumptions (or in case of higher consumptions).
David Hsu is also interested in visualizing buildings’ consumption data but from another point of view : “In 2009, laws had been passed in New-York City [such as the Local Law 84 then Local Law 87] which forced some buildings to make their consumption data available to the public.” In New-York’s case, this initiative is rather interesting as 1% of the buildings consumes nearly 55% of the overall city’s power. “I therefore analysed the effects these shared data had on the buildings’ power consumption. The results were quite surprising: 3 years after this regulation was passed, the power consumption of the city had decreased by 11%”. Two behavioural phenomenons can explain such result. On one hand, the possibility to follow one’s own power consumption leads to a moderating process from its users. Furthermore, having access to other buildings’ data creates a market effect (to remain competitive, buildings need to present satisfying power performances).
Visualizing these data does not require the use of AI. However, this technology can be used to understand and model the power consumption of buildings. Indeed, too often “buildings do not work the way we designed them to be” (D.H.). The use of consumption data could also help to create “buildings classification” (D.H.) with instances to follow for each classification (according to their power efficiency). The AI could therefore help identify patterns and present contextual examples from which a building could inspire itself (given its specific characteristics).
AI technology as a tool for green buildings
In 2030, 50% of buildings on the US soil would have been built in the years 2000. It is therefore critical to be able to optimize these existing buildings (with often ageing infrastructures). “Next, we would like to optimize the HVAC system (Heating, Ventilation, Air-Conditioning) of a building. We therefore believe we could diminish a building’s power consumption by 10% to 20%.” Beyond obvious (and yet efficient) actions which can be easily automated (switch off the lights, reduce the use of air-conditioning while nobody is in a room, etc.) Maxen Technology hopes to reach this goal by acting on complex data. Therefore, the startup company trains its AI to optimize a building’s power efficiency while taking external (weather conditions) and internal (a surface can benefit from the heat its downstairs floor produces) data into account. “We therefore want to go from a responsive model [turn the thermostat down when it’s too hot] to a proactive model” (S.N-P).
AI can also be used to increase the renewable energies used by the buildings. “In Texas, you can be paid, especially at night, to use the power the wind turbine produces” (D.H.). This phenomenon can be explained by a difference between a supply in green energies and a demand in energy. As a result, by foreseeing consumption peaks (and renewable energies production), the AI could solve the problem. Still in Texas “we could think about smart objects which would recharge themselves automatically at night [or during other time periods while renewable energy peaks are being produced]”.
However, and despite these possibilities, the AI is far from being a miracle solution. “We will still need to use non-renewable energies. Simply because the wind does not always blow or the sun does not shine all day long…” (D.H.). If this technology can optimize models, it is our responsibility to question them and to come up with others, more durable.