Opportunities of AI for monitoring nature in cities
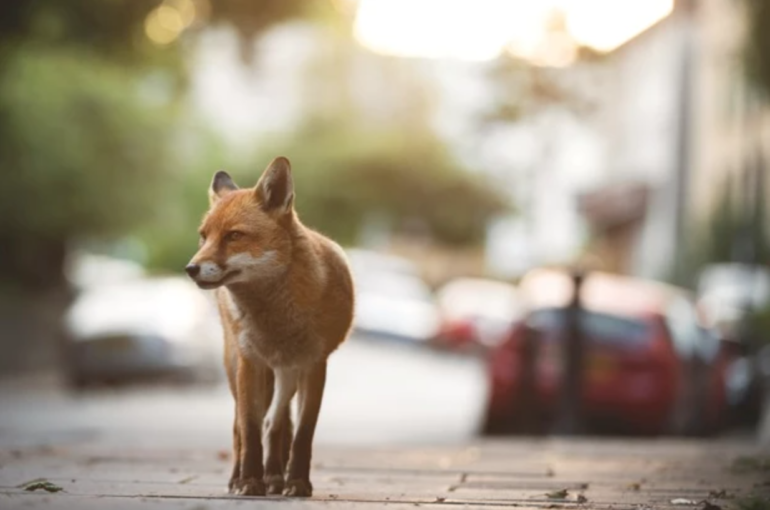
By Alison J.Fairbrass ( Institute for Sustainable Resources, University College London and Centre for Biodiversity and Environment Research, University College London)
Summary
Urbanisation drives the conversion of natural to built environments and causes the destruction and fragmentation of habitats on which wildlife depend. Cities can also provide novel and diverse habitats which act as refuges for wildlife unable to persist in intensively managed agricultural landscapes. The nature in cities provides a range of valuable ecosystem services to urban populations which may support human health and well-being. Monitoring nature is resource intensive and is complicated in cities by difficulties of access to private land and safety and security concerns for surveyors and equipment. Environmental sensing technology provides opportunities to overcome some of these difficulties and facilitates the generation of large volumes of environmental data. Artificial intelligence (AI) methods enable this data to be turned into meaningful information about nature with the development of algorithms for detecting and classifying the sounds made by, and images of, wildlife and the natural environment. Cities can support the development of autonomous urban nature monitoring systems by combining AI methods and sensors connected to Wifi and power in the form of Internet of Things (IoT) systems. Areas of future research include the development of AI algorithms for a wide range of species that live in cities, scaling up IoT systems to the city-scale, and informing city decision-making with these new information streams.
The importance of nature in cities
Urbanisation can have destructive effects on nature, driving the conversion of natural habitats into built environments, causing the destruction and fragmentation of the habitats on which wildlife depend1. Conversely, due to the heterogeneous nature of how the land in cities is managed, cities can also support diverse wildlife and habitats2, 3, in particular those species that can no longer survive in intensive agricultural environments4. More than half of the world’s population now live in cities5. Nature in cities can provide a wide range of valuable services to urban populations, including pest regulation, food production and air quality management6 which may benefit the health and well-being of urban populations7.
The current state of urban wildlife monitoring
Despite the potential importance of both cities for nature and the nature in cities for urban populations, there are few examples of systematic monitoring of the nature in cities. Wildlife is often difficult to monitor. It requires expertise to identify different wildlife species, often involves the use of technical equipment, and requires repeated surveys at multiple sites to monitor changes over time and space. In cities there are additional difficulties including access to private land and safety issues for surveyors and equipment8. Despite these difficulties, impressive datasets covering multiple species over several years have been compiled for a small number of cities. The Long-Term Ecological Research Projects in Baltimore and Phoenix, United States of America, have compiled datasets on birds, insects and plants in the cities since 19989, 10. The Urban Wildlife Information Network employs various monitoring methods, including camera traps, in cities following a shared protocol to produce long term data of terrestrial mammals from at least 24 cities to date11. This novel research structure departs from the traditional single-city approach by identifying the broad patterns of how wildlife respond and adapt to urbanization. These projects provide inspiring examples of how long-term monitoring of urban nature can be conducted.
New opportunities for urban wildlife monitoring
Environmental sensing technology, such as acoustic and camera sensors, is being increasingly used to collect information on wildlife and the natural environment12, 13. Sensors can be deployed for long time periods, making it possible to generate large audio, image and video datasets. Artificial intelligence (AI) methods offer exciting opportunities for turning this data into meaningful information about the nature and wildlife recorded using sensing technology. AI methods have been developed that can detect and classify the sounds and images of a range of wildlife species14, 15 as well as ecoacoustic measures of biotic sound that can provide proxy measures of biodiversity16. In cities, AI methods are being developed to process large urban nature datasets that are being generated using environmental sensing technology. The London HogWatch project is a large-scale urban camera trap survey focussed on urban mammals and their use of public green spaces and private gardens17. Both the London HogWatch project and the Urban Wildlife Information Network are using AI methods to process the vast amounts of images of urban mammals they are collecting.
Cities are the perfect environment in which to combine these new environmental sensing technologies and AI methods. The availability of power and internet networks means it is possible to develop Internet of Things (IoT) monitoring networks of connected sensors18. An example of such a system exists at the Olympic Park in London, United Kingdom, where a network of ultrasonic sensors are connected to the Park’s local Wifi network and powered through lampposts. Two AI algorithms on-board each of the 15 sensors process the ultrasonic recordings by first detecting bat calls, and then classifying calls to bat species, with results sent to a cloud server and reported in near-real-time on a public website (www.batslondon.com).
The next steps of this technology is the development of new and existing AI methods for detecting and classifying, in acoustic and image data, a wide range of species that inhabit cities. The autonomous bat monitoring system in London provides a proof of concept of an urban IoT network for wildlife monitoring and needs to be replicated at a city-scale to better understand the resource and capacity requirements of a city-scale autonomous urban nature monitoring system. Finally, we need to understand how to use these new sources of data and information to inform decision-making in cities about nature.
Alison J.Fairbrass
This contribution was originally written for URBAN AI Report
References
- Grimm NB, Faeth SH, Golubiewski NE, et al. Global change and the ecology of cities. Science 2008; 319: 756-760.
- Perring MP, Manning P, Hobbs RJ, et al. Novel Urban Ecosystems and Ecosystem Services. In: Hobbs RJ, Higgs ES and Hall CM (eds) Novel Ecosystems. Oxford: John Wiley & Sons, Ltd, 2013, pp.310-325.
- Aronson MFJ, La Sorte FA, Nilon CH, et al. A global analysis of the impacts of urbanization on bird and plant diversity reveals key anthropogenic drivers. 281(1780), http://rspb.royalsocietypublishing.org/content/281/1780/20133330.abstract (2014, accessed April 7, 2014).
- Hall DM, Camilo GR, Tonietto RK, et al. The city as a refuge for insect pollinators. Conservation Biology 2016; 31: 24-29.
- United Nations Department of Economic and Social Affairs. World Urbanization Prospects The 2018 Revision. 2019. New York: United Nations.
- Gómez-Baggethun E and Barton DN. Classifying and valuing ecosystem services for urban planning. Ecological Economics 2013; 86: 235–245. DOI: http://dx.doi.org/10.1016/j.ecolecon.2012.08.019.
- Marselle MR, Martens D, Dallimer M, et al. Review of the Mental Health and Well-being Benefits of Biodiversity. In: Marselle MR, Stadler J, Korn H, et al. (eds) Biodiversity and Health in the Face of Climate Change. Cham: Springer International Publishing, 2019, pp.175-211.
- Farinha-Marques P, Lameiras J, Fernandes C, et al. Urban biodiversity: a review of current concepts and contributions to multidisciplinary approaches. Innovation: The European Journal of Social Science Research 2011; 24: 247-271.
- Templeton LK, Neel MC, Groffman PM, et al. Changes in vegetation structure and composition of urban and rural forest patches in Baltimore from 1998 to 2015. Forest Ecology and Management 2019; 454: 117665. DOI: https://doi.org/10.1016/j.foreco.2019.117665.
- Central Arizona-Phoenix Long-Term Ecological Research CAP LTER. Long-Term Monitoring and Experiments, https://sustainability.asu.edu/caplter/research/long-term-monitoring/ (2020, accessed 22/01/2020).
- Magle SB, Fidino M, Lehrer EW, et al. Advancing urban wildlife research through a multi-city collaboration. Frontiers in Ecology and the Environment 2019; 17: 232-239. DOI: 10.1002/fee.2030.
- Gibb R, Browning E, Glover-Kapfer P, et al. Emerging opportunities and challenges for passive acoustics in ecological assessment and monitoring. Methods in Ecology and Evolution 2019; 10: 169-185. DOI: 10.1111/2041-210x.13101.
- Glover-Kapfer P, Soto-Navarro CA and Wearn OR. Camera-trapping version 3.0: current constraints and future priorities for development. Remote Sensing in Ecology and Conservation 2019; 5: 209-223. DOI: 10.1002/rse2.106.
- Christin S, Hervet É and Lecomte N. Applications for deep learning in ecology. Methods in Ecology and Evolution 2019; 10: 1632-1644. DOI: 10.1111/2041-210x.13256.
- Wäldchen J and Mäder P. Machine learning for image based species identification. Methods in Ecology and Evolution 2018; 9: 2216-2225. DOI: 10.1111/2041-210x.13075.
- Fairbrass AJ, Firman M, Williams C, et al. CityNet—Deep learning tools for urban ecoacoustic assessment. Methods in Ecology and Evolution 2019; 10: 186-197. DOI: 10.1111/2041-210x.13114.
- London Institute of Zoology. London HogWatch: Twitter Account, https://twitter.com/LondonHogWatch (2020, accessed 11/02/2020).
- Zanella A, Bui N, Castellani A, et al. Internet of things for smart cities. IEEE Internet of Things journal 2014; 1: 22-32.
Featured image from : https://www.discoverwildlife.com/how-to/photograph-wildlife/how-to-photograph-urban-wildlife/