Cognitive Analytics in Urban Science
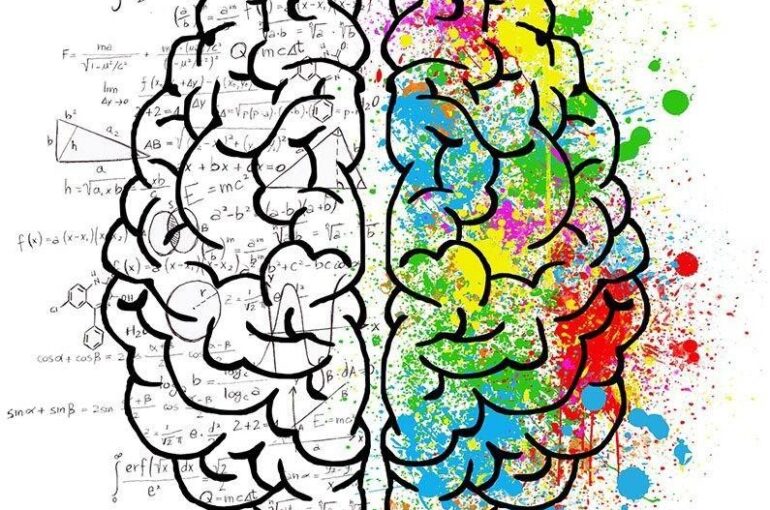
Laura Narvaez Zertuche is an Associate Partner at Foster + Partners. She is a registered Architect and MSc in Urban Design and Sustainable Development from Tec de Monterrey; she also holds a MSc and PhD in Architecture and the Built Environment from Space Syntax Laboratory, Bartlett School of Architecture UCL.
The journey of Data Science has evolved into different analytical approaches with the aim to answer different questions about the kind of data being created. Data comes in many ways: sensors used to gather climate information, social media sites, purchase transaction records, mobile phone GPS signals, or customer marketing trends to name a few. In spatial science, I often describe that if mobility and the way people move in cities was the lifeblood of the 20th century city, data is the very beating heart of the 21st century, making cities places of transactions [1].
In the same way cities have evolved to be places of transactions, the field of data science has also evolved its analytical perspectives to understand the built environment, from questioning what the data tells us (descriptive analytics), to ask why certain phenomena happens the way it does (diagnostic analytics), what could happen in the future (predictive analytics), and what actions can be taken next (prescriptive analytics). And now cognitive analytics, which relies on cognitive computing, has further strengthened the predictive power of machines by making them act like the human brain.
To understand what cognitive computing is designed to do, we can benefit from first understanding the quality it seeks to emulate: Cognitive intelligence. Cognitive intelligence is the human ability to think in abstract terms to reason, plan, create solutions to problems, and learn. Cognitive computing systems works in a similar way, like the human brain. They are able to process asynchronous information, to adapt and respond to events, and to carry out multiple cognitive processes simultaneously to solve a specific problem.
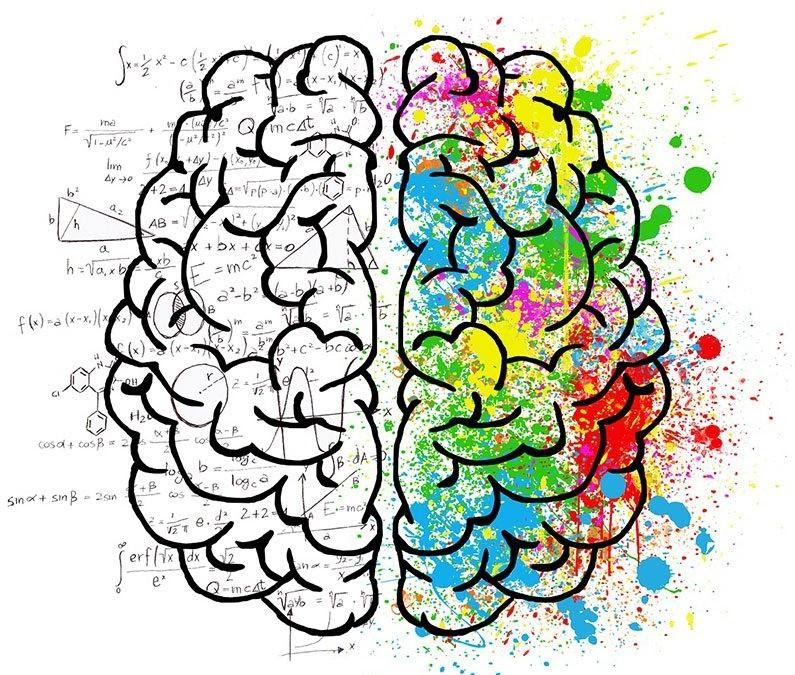
Available from: https://bold.expert/can-cognitive-training-really-make-us-smarter/
Whilst some experts see cognitive computing as a sub-field of Artificial Intelligence (AI) [2], others see the distinction differently. According to IBM, whilst AI powers machines to do human tasks, cognitive computing goes several steps further to create machines that can actually think like humans [3]. Another key difference lies in the way AI and cognitive computing are designed to tackled real-world problems. Whilst AI is meant to replace human intelligence, cognitive computing is meant to supplement and facilitate it.
In this article it is presented how cognitive analytics exemplifies the best possible blend of AI, machine learning (ML), deep learning (DL) and semantic technologies applied in three variants of urban science.
Applications in GIS
Cognitive analytics has made a lot of creative applications possible even within the field of spatial science. For example, one of them has been the intersection of GIS and AI. Esri GIS technology with the Microsoft Cognitive Toolkit (CNTK) have advanced in applying computer vision to geospatial analysis. Some of the deep learning use cases (image below) include:
Image classification used for pedestrian and traffic management planning, object detection for infrastructure mapping and feature extraction, semantic segmentation to create land cover classification layers, and instance segmentation to help reconstructing 3D buildings from lidar data [4].
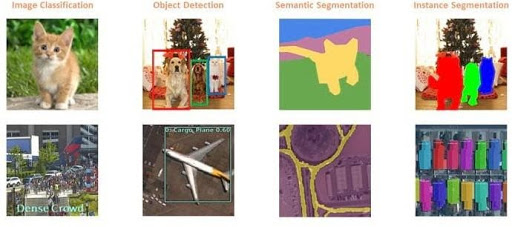
Available from: https://www.esri.com/about/newsroom/arcwatch/where-deep-learning-meets-gis/
Applications in Urban Research and Neuroscience
Another unique and different approach of combining semantic processing and urban analysis is a paper published in Nature, linking formal analysis of the street network and brain activation during navigation [5]. The research uses fMRI and a simulation of London (using MATLAB Cogent2000 Toolbox, semantics processing) including 26 streets as their testing area.
One of the ways to measure how and when the brain processes topological structures to guide future behaviour during everyday life was to combine the use of fMRI technology with Space Syntax methods (graph-theoretic analysis), which relate human behaviour to the spatial layout of the built environment.
Space Syntax methods provided a formal way of analysing the topological properties of the street network applied at different scales (e.g. local or global). This meant measuring properties of centrality in the selected 26 streets and how these are examined in relation to the fMRI analysis.
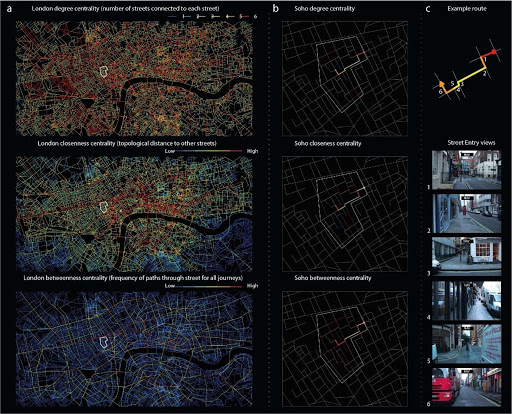
Published in: Javadi, AH., Emo, B., Howard, L. et al. Hippocampal and prefrontal processing of network topology to simulate the future. Nat Commun 8, 14652 (2017).
The image below shows an example of the study when new streets are entered during navigation of the city, showing right posterior hippocampal activity indexes the change in the number of local topological connections available for future travel and right anterior hippocampal activity reflects global properties of the street entered.
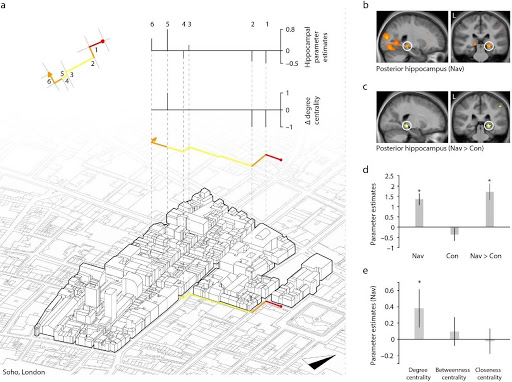
Published in: Javadi, AH., Emo, B., Howard, L. et al. Hippocampal and prefrontal processing of network topology to simulate the future. Nat Commun 8, 14652 (2017).
Applications in Urban Design Computing
The third application of cognitive analytics is the Cognitive Design Computing (CoDeC) [6] workstream of the Big Data Informed Urban Design and Governance project at the Future Cities Lab in Singapore in 2018. The idea of CoDeC was “to combine unique human design competences with computational methods for the generation, analysis, and exploration of urban designs”. Such computational methods enable an urban designer to approach a computational planning process from the perspective of what performance design solution can be achieved by generating a set of possible solutions.
The cognitive urban design computing system integrates available simulation methods and combines them with optimization and machine learning approaches, with the future aim being to mimic the was a designer’s brain works. “Given the complex nature of urban design problems, an additional aspect is the provision of models for human-computation interaction. Since urban design tasks cannot be fully automated, the computational burden of an urban design problem needs to be distributed between computer and designer.”
The workstream argues that one of the ways in which digital tools can support designers is by generating design proposals. Learning how designers use such computational design support systems, in combination with machine learning methods, it may be possible to derive to more complex artificial solution strategies that can help computers make more efficient suggestions in the future.
Below is an extract of the automatization of the spatial synthesis process, using multi-criteria optimization algorithms. For full information about the design space exploration methods, please visit: https://youtu.be/A79UNp9pAr4
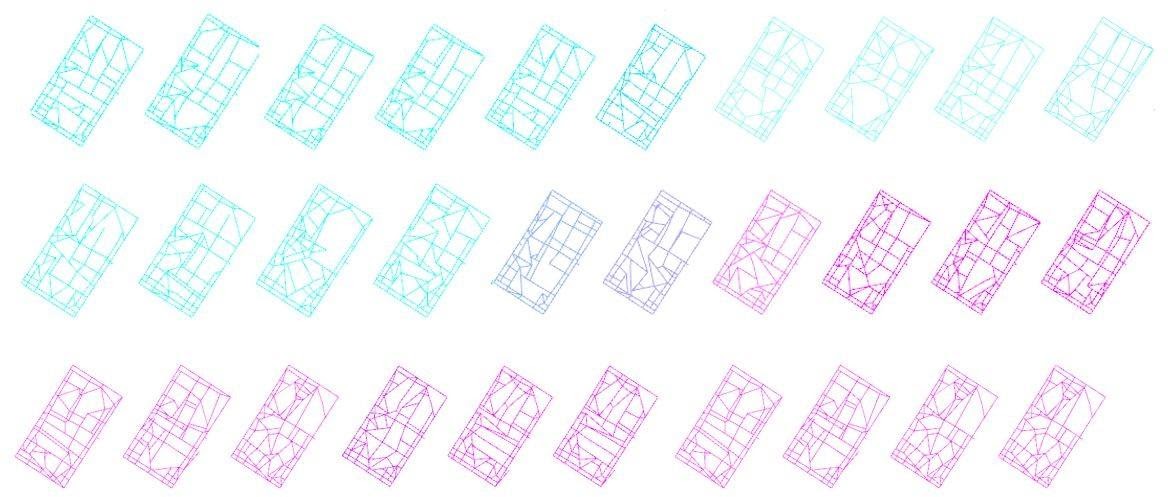
Available from: https://toolbox.decodingspaces.net/cognitive-urban-design-computing-fcl/
The three applications of cognitive analytics reveal how much potential this evolving approach in data science has had in urban technologies and research. In the GIS world, deep learning is rapidly evolving allowing data scientists to leverage cutting-edge research whilst taking advantage of industrial-strength GIS applications. The latest ArcGIS API for Python workflow has emerged from these deep learning capabilities. On the other hand, Neuroscience has played a key role in the evolution of AI systems. The interdisciplinary research of combining spatial cognition attributes with the use of AI systems, either in spatial analytics or computer science, are certainly ways to keep the journey of Data Science evolving to new and innovative analytical approaches.
Laura Narvaez Zertuche
References
[1] Sambiasi, S. 7 Architectural Considerations that are Shaping Future Cities (2019), ArchDaily. Available from: https://www.archdaily.com/927262/7-architectural-considerations-that-are-shaping-future-cities [Accessed: 9 February 2021].[2] Roe, C. A Brief History of Cognitive Computing (2014), Dataversity. Available from: https://www.dataversity.net/brief-history-cognitive-computing/#:~:text=The%20first%20underpinnings%20of%20modern,was%20coined%20by%20the%2 0late [Accessed: 11 February 2021].
[3] Schroeer, T. Cognitive computing: Hello Watson on the shop floor (2017), IBM. Available from: https://www.ibm.com/blogs/internet-of-things/iot-cognitive-computing-watson/#:~:text=Cognitive%20computing%20refers%20to%20systems,their%20experiences%20with%2 0their%20environment [Accessed: 11 February 2021].
[4] Singh, R. Where Deep Learning Meets GIS (2019), ESRI. Available from : https://www.esri.com/about/newsroom/arcwatch/where-deep-learning-meets-gis/ [Accessed: 11 February 2021].
[5] Javadi, AH., Emo, B., Howard, L. et al. Hippocampal and prefrontal processing of network topology to simulate the future. Nat Commun 8, 14652 (2017). https://doi.org/10.1038/ncomms14652
[6] The Virtual and the Real in Planning and Urban Design: Perspectives, Practices and Applications (pp.288) Chapter: 2.1. (2017), Routledge. Editors: Claudia Yamu, Alenka Poplin, Oswald Devisch, Gert De Roo. Also available from: https://toolbox.decodingspaces.net/cognitive-urban-design-computing-fcl/ [Accessed: 13 February 2021].